Applying Deep Learning to Localization Microscopy

Modern science requires modern technological solutions. As we prise the natural world apart in search of answers to ever more complex questions, we need to be thinking in new ways about our approach to the problems we are faced with.
Image Credit: Archy13/Shutterstock.com
Several technologies have been developed over the past few years that are pushing the boundaries of our scientific knowledge to new heights. As these technologies develop scientists are looking into ways of using them in tandem, to produce more accurate results and new ways of approaching the problems of the modern scientific industry. Two such technologies that can be combined to produce a better understanding of biological systems are localization microscopy and deep learning.
Localization microscopy
Localization microscopy is a recently developed technique that has the potential to transform our understanding of cell function at the molecular level. It provides pin-point accurate information about molecular distributions in biological structures, and analysis with this technique can even provide the absolute number of proteins present in biological systems.
It is a powerful high-resolution imagining technique that has been shown to have the potential to be an extremely useful complementary method for experiments, utilizing conventional light and electron microscopy. This technique provides direct experimental feedback for modeling the complexity of biological interactions.
Localization microscopy works on the principle that the diffraction barrier (the resolution limit of light waves) can be bypassed by photoswitching, photoactivation, or photoconversion of individual fluorophores, which are attached to the structure of interest.
By utilizing precise single-molecule localization in conjunction with advanced reconstruction techniques of multiple images, which contain a handful of active isolated fluorophores, structures of less than half the wavelength of light in diameter can be resolved, compared with techniques such as conventional or confocal fluorescence microscopy. The density of activated molecules is kept low so that the individual fluorophores being imaged in each cycle do not overlap.
Thus, using this technique, detection of a mere few thousand protons from a single fluorophore allows localization of said fluorophore with precision in the nanometer range.
Applying deep learning to produce better, faster results
However, there is one limitation with localization microscopy: the number of images that can be produced in a given time. As the technology relies on multiple images of different activated fluorophores, this is a major stumbling block without the application of other technologies to speed up the process. One such current technology that can resolve this issue is deep learning.
In the field of artificial intelligence (AI), deep learning is a recently developed subset of machine learning. It utilizes artificial neural networks to make it capable of learning from unstructured or unlabelled data. It has been used in many different applications from facial recognition to self-driving cars, and healthcare applications. It is a cutting-edge technology that is fundamentally changing how software and machines interact with the world around us.
Recently, studies have been carried out by researchers to apply deep learning to life science research and complement the advances within the field. One such study that sought to provide better and faster localization microscopy high-throughput images was carried out by a team led by Wei Ouyang, of the Imaging and Modeling unit of the Pasteur Institute in Paris. They developed a system called ANNA-PALM, which was shown to vastly improve super-resolution images of delicate structures.
They used simulations and experimental imaging of mitochondria, microtubules, and nuclear pores to demonstrate that this technology could reconstruct high-quality super-resolution images from up to two orders of magnitude fewer frames than would normally be needed, without compromising spatial resolution.
With ANNA-PALM, they managed to achieve super-resolution reconstruction of widefield images alone (a difficult proposition without the addition of deep learning techniques), and by adding localization data image quality was improved. The system yielded images spanning spatial scales from ~20nm – ~2nm and imaged >1,000 fields of view containing >1,000 cells in ~3h. Acquisition time and sample irradiation were drastically reduced, leading to gentler and faster high-throughput and live-cell super-resolution imaging.
The future
By utilizing cutting-edge technologies, researchers can easily complement existing techniques to produce results that are faster and more accurate than ever before. Through applications such as improving localization microscopy imaging, deep learning is one such technology that is advancing the way we learn about the complexities of the natural world and represents a revolutionary development in current and future scientific studies.
Sources
Sauer, M (2013) Localization microscopy coming of age: from concepts to biological impact, Journal of Cell Science 126 pgs. 3505-3513 DOI: https://doi.org/10.1242/jcs.123612
Ouyang, W et al. (2018) Deep learning massively accelerates super-resolution localization microscopy, Nature Biotechnology 36, pgs. 460–468 DOI: https://doi.org/10.1038/nbt.4106
ANNA-PALM, Institut Pasteur https://annapalm.pasteur.fr/#/
Last Updated: Jan 17, 2020
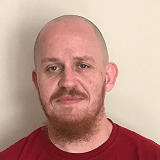
Written by
Reginald Davey
Reg Davey is a freelance copywriter and editor based in Nottingham in the United Kingdom. Writing for News Medical represents the coming together of various interests and fields he has been interested and involved in over the years, including Microbiology, Biomedical Sciences, and Environmental Science.
Source: Read Full Article