SARS-CoV-2 detection by machine learning surface-enhanced Raman spectroscopy

Surface-enhanced Raman spectroscopy is a highly sensitive molecule characterization method, significantly more sensitive than ordinary Raman spectroscopy due to the excitation of Raman modes matching the plasmon resonance frequency of the surface metal utilized, usually gold, silver, or copper.
Molecules are immobilized on the surface of the metal, and in the case of DNA, the method is capable of nucleotide level resolution. The nanostructure of the surface dictates the wavelength of plasmon resonance of the gold surface, and in a paper recently uploaded to the preprint server medRxiv* by Torun et al. (August 8th, 2021), computational methods are used to design surfaces optimized for detecting severe acute respiratory syndrome coronavirus 2 (SARS-CoV-2).
Subsequently, machine learning was utilized to interpret the dataset, potentially creating a fast and highly accurate COVID-19 detector capable of distinguishing between variants of concern.
Designing the sensor
The sensor designed by the group consists of an 80 nm square silicon base with a 20 nm thick gold nanostructure coated in thiol-modified primary DNA aptamers, as the thiol-Au bond is strong and stable. Saliva samples collected from individuals suspected of having COVID-19 are mixed with Cy5.5-modified fluorescent secondary aptamers, with excitation and emission wavelengths of 678 and 694 nm, respectively. The sample is then applied to the sensor. The sensor is then excited using a 633 nm laser, and Raman shift spectra within 548–1620 cm-1 measured.
The primary DNA aptamers utilized were SARS-CoV-2 spike protein-specific. In contrast, the secondary aptamers were specific to viral glycoproteins, and thus when both bound to the virus, they create a sandwich assay intended to intensify the signal.
The researchers from Turkey and the US used computational methods to screen for and optimize the pattern of the gold nanostructure on the sensor, where calculated electric field intensity was the aim of enhancements. The group developed an algorithm that mutates the existing optimum surface iteratively, with the top 20% of the current "generation" selected to pass on their features.
The structures are mated over 30 generations, with descendants inheriting features along with a restricted set of mutations. The generated nanosensors were fabricated by lithography at Sabanci University and then functionalized with the thiol-aptamer.
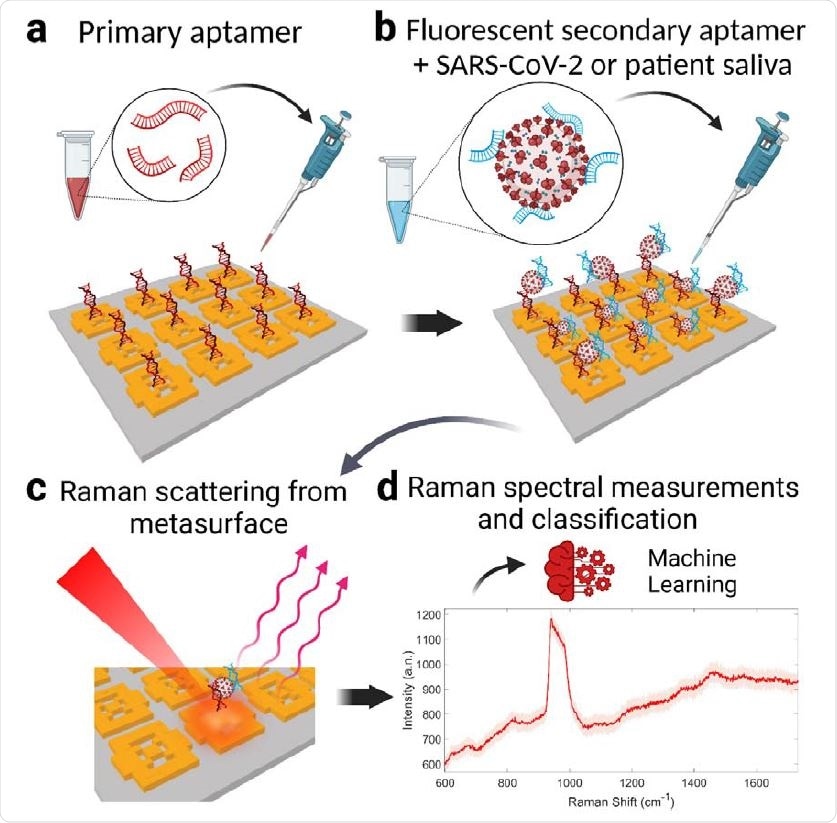
Testing the sensor
The specificity of the sensor was demonstrated by exposing the chip to Cy5.5-tagged SARS-CoV-2, human rhinovirus 1B, or human coronavirus 229E, with each being easily distinguished by differing spectral fingerprints.
Nasopharyngeal and saliva samples from 36 COVID-positive patients by PCR were obtained, along with samples from 33 healthy individuals tested by PCR and found to be negative. Raman spectra were collected at several hundred points on the chip, each representing around 1,000 data points.
The group state that the sensor achieved 99% sensitivity and specificity in viral presence identification, dropping to 95.2% when using unprocessed saliva. The Raman spectral data obtained were interpreted by machine learning. There were far too many data points to be assigned manually. Therefore, some of the datasets were used to "train" the machine before the remainder of the data was fed.
The collection of nasopharyngeal swabs requires trained staff and is unpleasant upon repeated testing, and the subsequent PCR testing often takes several days.
Alternatives such as lateral flow tests lack sensitivity in cases of low viral load, though such cases are still important to detect as these individuals may still be contagious. Some types of Raman spectrometer are hand-held, easily small enough to be deployed in the field.
Combined with the methods explored in this paper would provide results much more quickly, accurately, and reliably than PCR or lateral flow tests.
*Important Notice
medRxiv publishes preliminary scientific reports that are not peer-reviewed and, therefore, should not be regarded as conclusive, guide clinical practice/health-related behavior, or treated as established information.
- Torun, H. et al. (2021) "Machine learning detects SARS-CoV-2 and variants rapidly on DNA aptamer metasurfaces". medRxiv. doi: https://doi.org/10.1101/2021.08.07.21261749, https://www.medrxiv.org/content/10.1101/2021.08.07.21261749v1
Posted in: Device / Technology News | Medical Research News | Disease/Infection News
Tags: Aptamers, Assay, Biosensor, CHIP, Copper, Coronavirus, Coronavirus Disease COVID-19, DNA, Frequency, Lithography, Machine Learning, Molecule, Nucleotide, Protein, Raman Spectroscopy, Respiratory, Rhinovirus, SARS, SARS-CoV-2, Severe Acute Respiratory, Severe Acute Respiratory Syndrome, Spectrometer, Spectroscopy, Spike Protein, Syndrome, Virus, Wavelength
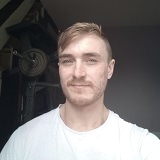
Written by
Michael Greenwood
Michael graduated from Manchester Metropolitan University with a B.Sc. in Chemistry in 2014, where he majored in organic, inorganic, physical and analytical chemistry. He is currently completing a Ph.D. on the design and production of gold nanoparticles able to act as multimodal anticancer agents, being both drug delivery platforms and radiation dose enhancers.
Source: Read Full Article